55. Rohin Shah - Effective altruism, AI safety, and learning human preferences from the state of the world
Towards Data Science - Un pódcast de The TDS team
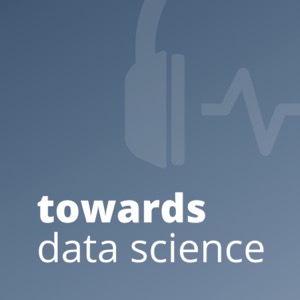
Categorías:
If you walked into a room filled with objects that were scattered around somewhat randomly, how important or expensive would you assume those objects were?
What if you walked into the same room, and instead found those objects carefully arranged in a very specific configuration that was unlikely to happen by chance?
These two scenarios hint at something important: human beings have shaped our environments in ways that reflect what we value. You might just learn more about what I value by taking a 10 minute stroll through my apartment than by spending 30 minutes talking to me as I try to put my life philosophy into words.
And that’s a pretty important idea, because as it turns out, one of the most important challenges in advanced AI today is finding ways to communicate our values to machines. If our environments implicitly encode part of our value system, then we might be able to teach machines to observe it, and learn about our preferences without our having to express them explicitly.
The idea of leveraging deriving human values from the state of an human-inhabited environment was first developed in a paper co-authored by Berkeley PhD and incoming DeepMind researcher Rohin Shah. Rohin has spent the last several years working on AI safety, and publishes the widely read AI alignment newsletter — and he was kind enough to join us for this episode of the Towards Data Science podcast, where we discussed his approach to AI safety, and his thoughts on risk mitigation strategies for advanced AI systems.